Mar 14, 2024PRESS RELEASE
Study results accepted by CVPR 2024, a top international conference on AI and computer vision
- Rikkyo researchers achieve greatly improved explainable AI with modern convolutional neural network -
Keyword:RESEARCH
OBJECTIVE.
A paper by Shunsuke Yasuki, a first-year doctoral student, and Associate Professor Masato Taki at the Graduate School of Artificial Intelligence and Science, Rikkyo University, on study results achieving high-quality explainable artificial intelligence has been accepted by CVPR 2024 (The IEEE/CVF Conference on Computer Vision and Pattern Recognition 2024). Details of the study results will be presented at the conference, which will be held in Seattle June 17-21, 2024.
CVPR is one of the most important conferences in the field of pattern recognition and computer vision. CVPR ranks fourth, following Nature, NEJM, and Science, in Google Scholar’s h5-index ranking for academic journals and international conferences in all scientific fields.
Research outlines
Improving the explainability regarding the basis for decisions made by artificial intelligence (AI) systems is an important issue in the field of AI. When AI makes decisions about images, especially in systems used for images, explainable algorithms that determine important image regions highlighted by AI are widely used. Starting from an older explainable algorithm called CAM (class activation mapping), many complex explainable algorithms have been proposed. In this study, it has been found that the use of knowledge regarding the latest image AI models, when simply combined with the most classic CAM, can achieve explicability whose quality is comparable to that of well-designed, state-of-the-art algorithms. Hence, this paper is titled “CAM Back Again.”
Research background
Since around 2012, deep learning using convolutional neural networks (CNNs) has made great progress. This has significantly advanced the computer vision field. In the past several years, inspired by the success of Transformer architectures such as ChatGPT, the computer vision field has been using Transformers, rather than CNNs, and their high performance has been in the spotlight. Since CNNs are inferior to Transformers in terms of performance, research has been actively conducted to narrow the performance gap between the two architectures, attracting attention to modern CNNs with larger kernel sizes (large kernel CNNs), among others. Large kernel CNNs have demonstrated high performance in a wide range of visual tasks such as object detection and segmentation. Prior research had attributed this high performance to large effective receptive fields obtained via large kernels. However, there was no sufficient evidence to support this view. Our research therefore examined the validity of this non-trivial view in terms of a visual task called WSOL (weakly supervised object localization).
WSOL uses AI models for image classification to determine not only classes, but also the location of an object in the image. CAM is a well-known, classic method for this task. CAM is designed to pinpoint the actual object region in the image as the basis for prediction in terms of image classification by AI. In practice, however, CAM tends to extract only a portion of the object, rather than the entire object, as the basis for prediction. For example, at the top of the figure below, only the face of the bird is in focus. Thus, CAM is not capable of accurately localizing the entire object. To solve this problem, a large number of ingeniously devised improved algorithms have been proposed.
WSOL uses AI models for image classification to determine not only classes, but also the location of an object in the image. CAM is a well-known, classic method for this task. CAM is designed to pinpoint the actual object region in the image as the basis for prediction in terms of image classification by AI. In practice, however, CAM tends to extract only a portion of the object, rather than the entire object, as the basis for prediction. For example, at the top of the figure below, only the face of the bird is in focus. Thus, CAM is not capable of accurately localizing the entire object. To solve this problem, a large number of ingeniously devised improved algorithms have been proposed.
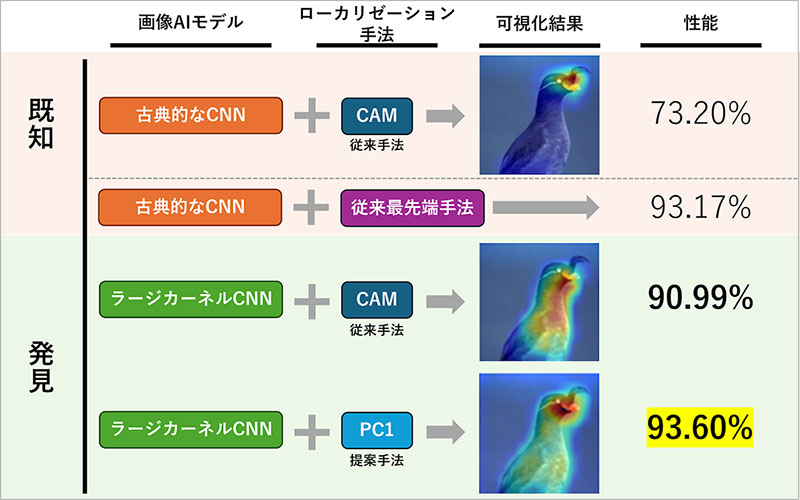
Comparison of traditional WSOL method and proposed method
Results
In this study, it has been found that large kernel CNNs exhibit high WSOL performance (localization performance) and the factors contributing to this performance have been thoroughly analyzed. First, to verify the validity of the existing view, whether the effective receptive field size improves WSOL performance was investigated. The results presented many experimental findings that do not support the existing view that effective receptive fields lead to improved performance.
The study then reported many findings from the analysis. First, it has been found that modern large kernel CNNs automatically resolve the problems with traditional CAMs. Since the launch of CAMs in 2016, many methods have been proposed to improve their performance. This study has obtained WSOL performance that outperforms that by most of the numerous previous studies simply by combining large kernel CNN and CAM. This is because large kernel CNNs are inherently able to capture the adequate amount of global information of image features.
In addition, this study has found that objects can be precisely localized simply by performing first principal component analysis (PC1) on image features. With this new method, the most advanced performance of WSOL using CNN has been achieved. Based on these findings, we have concluded that the state-of-the-art performance of large kernel CNNs on a variety of tasks is due to the inherent capabilities of the architecture and the resulting improvement in image features.
The study then reported many findings from the analysis. First, it has been found that modern large kernel CNNs automatically resolve the problems with traditional CAMs. Since the launch of CAMs in 2016, many methods have been proposed to improve their performance. This study has obtained WSOL performance that outperforms that by most of the numerous previous studies simply by combining large kernel CNN and CAM. This is because large kernel CNNs are inherently able to capture the adequate amount of global information of image features.
In addition, this study has found that objects can be precisely localized simply by performing first principal component analysis (PC1) on image features. With this new method, the most advanced performance of WSOL using CNN has been achieved. Based on these findings, we have concluded that the state-of-the-art performance of large kernel CNNs on a variety of tasks is due to the inherent capabilities of the architecture and the resulting improvement in image features.
Future prospects
Society has high expectations for explainable algorithms as a method to increase the transparency of AI. In reality, however, existing explainable algorithms do not provide sufficiently reliable results, as explained results vary widely from method to method. This study shows that improving the performance of latest image AI models can result in significantly improving explanation results obtained from long-used explicable algorithms. This finding elucidates the behavior of explicable algorithms and makes operations by transparent AI more feasible. Once highly reliable AI is developed, it can be used for a wide range of applications, such as extracting scientific knowledge that humans do not currently possess or directly learning the knowledge the AI has acquired from data. We will continue our research with the goal of developing AI applications that can be useful in such deeper aspects of human society.
Keywords
- Convolution: A method for extracting information from images, etc., by aggregating local information; a kind of filtering
- Convolutional neural network: A neural network that uses the convolution operation and is dedicated to images
- Kernel size: The size of the area where local information is collected for aggregation by convolution
- CAM (class activation mapping): It visualizes the region corresponding to the basis for determining that the inferred image belongs to the predicted class by operations using the weight of the classification layer of the image classification model and the features of the inference process.
- WSOL (weakly supervised object localization): A task that causes AI that has only learned image classification to even determine the location and area of a classified object. It is called “weakly supervised” in the sense that it learns classification, but not location information.
- Effective receptive field: A kind of “field view” of an AI model
- Feature: A variable that serves as a clue to prediction by AI. In deep learning, AI itself discovers features.
- Computer vision: The field of computer image recognition and processing
- Transformer: A neural network capable of global information aggregation
- Principal component analysis: A method for summarizing information on multivariate data and transforming it into data with fewer variables that represent the essence of the original data
Article Information
- Title: CAM Back Again: Large Kernel CNNs from a Weakly Supervised Object Localization Perspective
- Authors: Shunsuke Yasuki, Masato Taki
- Link: https://arxiv.org/abs/2403.06676
Taki Laboratory headed by Masato Taki at the Graduate School of Artificial Intelligence and Science
Taki Laboratory conducts extensive research on deep learning, from fundamentals to applications, that will support future applications of artificial intelligence. In addition to the study results discussed in this article, the laboratory has produced various study results, including the papers accepted respectively by NeurIPS 2022 and AAAI 2024, which are international conferences in the field of AI and machine learning.