Feb 27, 2023PRESS RELEASE
Researchers develops example-based method to explain AI inference results
Keyword:RESEARCH
OBJECTIVE.
A team of researchers has succeeded in developing a method of explaining how an artificial intelligence (AI) model has made a decision, or explainable AI. The method enables verification of the reliability of AI inferences in a simple and intuitive manner.
The research team includes Specially Appointed Associate Professor Shin-nosuke Ishikawa and Associate Professor Masato Taki and Professor Yasunobu Uchiyama of Rikkyo University’s Graduate School of Artificial Intelligence and Science. Mamezou Co., Ltd., a Tokyo-based IT consulting firm headed by Tetsuya Nakahara, also joined the team. An article summarizing the research results was published in the International Journal of Applied Earth Observation and Geoinformation in April 2023.
Background
- High expectations placed on explainable AI
Thanks to the rapid advancement of AI technology in recent years, increasing attention is being paid to it as a way of dealing with various social issues. But there is some hesitance about introducing AI due to doubts concerning the reliability of AI inference results. To solve this problem, research is being conducted into explainable AI (XAI), which is capable of explaining and interpreting AI inference results. It is helpful to understand how a certain model’s inferences have been derived. The AI Utilization Guidelines formulated by the Institute for Information and Communications Policy under the Ministry of Internal Affairs and Communications say that AI service providers should pay attention to the explicability of AI judgments in accordance with the principle of transparency.
Extracting factors that are considered to have had a major influence on AI inference results is a typical explainable AI technique. For example, if AI inference results show that you have a high risk of becoming ill, the technique explains that your high blood pressure level is a major factor. This technique helps a person’s intuitive understanding, but its easy-to-understand nature can compromise the rich expressive power of sophisticated AI models. This is because the technique employs unrealistic hypotheses or simplistic approaches. This study, in contrast, takes a different approach to explain AI inference results.
- Data-centric perspective
Whether AI can accurately make inferences depends on an AI model structure or training algorithms, as well as which dataset was used to train the model. In recent years, it has been pointed out that the use of AI should be considered from a data-centric perspective. The reason is that, in many cases, it has become important to improve the quantity and quality of dataset for training—rather than using complicated models—if the accuracy of AI is to be enhanced for practical use in society. So far, many methods of explainable AI focus on process, such as how inferences are derived from input data, while virtually no method focused on the training dataset.
Putting aside AI, how do we normally determine if certain judgments are reliable? For example, let’s say some people have decided a certain object is a cat. To verify this opinion, do people seek explanations with the regard to the cognitive judgment process, such as “the light that reflects the cat is captured by their eyes,” or “electric signals stimulate their brain cells”? A more persuasive explanation is that animals they have seen before are the basis of the inference. The judgment of a person who has never seen a cat is not so reliable. If people have a cat similar to the image shown, their judgment seems to be reliable. This is a data-centric perspective, based on previous experience.
Research results
- WIK: A method to show from which dataset AI learned
By using a data-centric approach, this study succeeded in developing a method of explainable AI that explains inferences made by AI models by showing actual examples of data that were used to train AI models. This method shows which one an AI model has chosen as the most similar to the input dataset among the datasets on which it was trained. The team named the method as “What I know (WIK).”
Several approaches similar to the team’s method have been proposed. But the team’s method is different in that it considers the final decision an AI model made when presenting actual examples. When verifying the reliability of an AI inference that an object is a cat, what we want to know seems to be which object is most similar to cats that you have seen in the past, or what is most similar to the object you are now seeing. If the AI model determines it is a cat, but also similar to a loaf of bread that it has seen before, it is meaningless to verify what is a confusing inference. Therefore, in this method, the team used only examples of training datasets that are similar to the input data to be inferred.
- Confirming the method’s reliability in classifying satellite dataset
In the article, the team demonstrated its reliability in classifying images of Earth as observed by a satellite. A deep-learning model adopted by the team classifies what was captured in the images into 10 categories, such as “forest,” “residential,” “river” and “highway.” Five types of satellite image datasets were adopted for classification. WIK then presented images similar to the input data, as shown in the figure below. The top row of images corresponds to the target data to be classified. The bottom row shows images that were determined to be similar to the images in the top row. Since the images in the bottom row are similar to the ones above, it can be said that this model has been trained sufficiently to classify the images in the top row. (Or, it has sufficient “experience,” as in the cat example). This shows that this model’s inference results can be reliable.
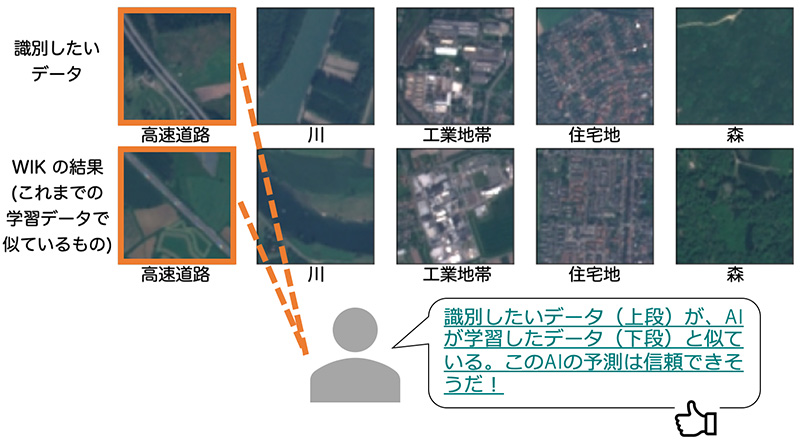
Diagram showing an example of how the proposed method can verify the reliability of a deep-learning model classifying a satellite dataset.
Future prospects
Article information
Article link: https://doi.org/10.1016/j.jag.2023.103215
arXiv: https://arxiv.org/abs/2302.01526